GeneGPT: Revolutionizing Genomics Question Answering with Artificial Intelligence
In recent years, large language models (LLMs) have emerged as powerful tools for processing and interpreting vast amounts of data. These models have proven instrumental in various domains, including genomics, where they have played a crucial role in answering complex questions and facilitating research. However, LLMs often face challenges in retrieving precise and accurate information from specialized fields like genomics. This is where GeneGPT, a novel artificial intelligence method, comes into play.
Bridging the Gap between LLMs and Genomic Databases
One of the major limitations of LLMs is their inability to directly consult verified sources of information, such as biomedical databases. This limitation can lead to the generation of plausible yet inaccurate responses. To address this challenge, researchers have developed GeneGPT, a groundbreaking methodology that bridges the gap between LLMs and the National Center for Biotechnology Information (NCBI) databases.
The core idea behind GeneGPT is to augment LLMs with the ability to access and interpret data from specialized sources, specifically the web APIs of the NCBI. By integrating LLMs with these APIs, GeneGPT enables the models to perform targeted searches and retrieve information directly from NCBI’s databases, ensuring access to the most current and relevant genomic data.
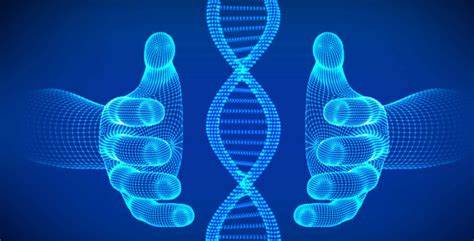
Unleashing the Power of GeneGPT
GeneGPT’s methodology involves training LLMs to generate and execute API calls to NCBI’s web APIs effectively. This is achieved through in-context learning and a specialized decoding algorithm that recognizes and acts upon these API requests. By enabling LLMs to directly access NCBI’s databases, GeneGPT ensures that the information retrieved is current and highly relevant to the user’s query.
Notably, GeneGPT outperforms existing models and methodologies in terms of accuracy and efficiency in retrieving biomedical information. It excels in handling complex, multi-hop questions that require sequential API calls, showcasing its ability to navigate through a series of interconnected queries to arrive at a precise answer.
Advancing Biomedical Research and Beyond
The success of GeneGPT extends beyond the field of genomics. By bridging the gap between LLMs and specialized databases, GeneGPT addresses the challenge of inaccurate information retrieval, opening up new possibilities for leveraging LLMs in tasks that require access to specific, verified knowledge. This advancement promises to make LLMs more versatile and reliable tools for researchers and professionals across various domains.
Furthermore, GeneGPT’s success highlights the transformative potential of augmented LLMs in navigating the vast and complex landscape of biomedical knowledge. By enabling LLMs to access and utilize specialized knowledge from NCBI’s databases directly, GeneGPT marks a significant leap forward in enhancing the capabilities of LLMs in biomedical research. This development paves the way for further innovations in the application of artificial intelligence in biomedical research and beyond.
Conclusion
GeneGPT represents a revolutionary approach to enhance the capabilities of LLMs for answering genomics questions. By integrating LLMs with the web APIs of the NCBI, GeneGPT enables direct access to specialized knowledge and ensures retrieval of up-to-date and accurate information. This breakthrough methodology addresses the limitations of traditional database queries and opens up new possibilities for leveraging LLMs in genomics research and beyond.
The success of GeneGPT highlights the power of artificial intelligence in transforming the way we approach biomedical research. As LLMs continue to evolve and integrate with domain-specific tools, we can expect further advancements in information retrieval and knowledge generation. GeneGPT is just the beginning of a new era in genomics question answering, bringing us closer to unlocking the full potential of artificial intelligence in the biomedical field.
Check out the Paper and Github. All credit for this research goes to the researchers of this project. Also, don’t forget to follow us on LinkedIn. Do join our active AI community on Discord.
If you like our work, you will love our Newsletter 📰