Enhancing AI Validation with Causal Chambers: Bridging Data Gaps in Machine Learning and Statistics with Controlled Environments
Artificial intelligence (AI) and machine learning (ML) have revolutionized various industries, enabling computers to perform complex tasks and make predictions with remarkable accuracy. However, the effectiveness and reliability of AI models heavily rely on the availability of high-quality, real-world data for validation. Without access to comprehensive and diverse datasets, the performance of these models may suffer when applied to real-world scenarios.
One of the key challenges in AI research is the reliance on synthetic data, which is often unable to fully capture the complexities and variability of natural environments. AI models trained and tested under ideal conditions with simple or tailored datasets may not effectively handle the uncertainties and challenges present in real-world settings. Therefore, there is a need for innovative solutions that bridge the gap between theoretical AI models and practical applications.
To address this challenge, a team of statisticians from ETH Zurich has developed a groundbreaking solution called “causal chambers”. Causal chambers are controlled environments that can simulate and manipulate various physical phenomena, allowing for the generation of diverse data types, including time series and image data. These chambers serve as a bridge between simulated datasets and real-world data, providing a ground truth for validating AI methodologies in areas where suitable datasets are otherwise unavailable.
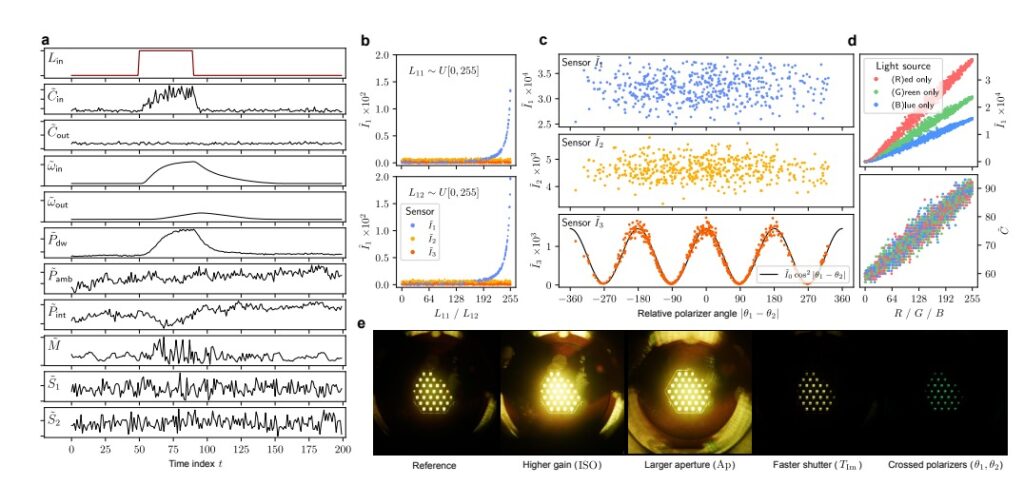
Equipped with sensors and actuators, causal chambers can generate vast datasets from relatively simple physical systems. The chambers can manipulate variables such as light intensity, air pressure, and mechanical component positions, creating controlled conditions that test the robustness and applicability of AI models. By producing reliable and real-world applicable data, causal chambers enable researchers to validate AI models in a controlled environment before deploying them in real-world scenarios.
The utility of causal chambers is demonstrated across various AI domains. In causal discovery, researchers can meticulously perform interventions and observe the outcomes, allowing for the empirical validation of causal models generated by AI systems. This capability is particularly valuable in domains where causality plays a crucial role, such as healthcare and finance.
Furthermore, causal chambers are instrumental in tasks like symbolic regression, where they help reveal underlying mathematical relationships within the data, similar to discovering natural laws. By integrating symbolic regression with causal chambers, researchers can uncover hidden patterns and relationships that may have otherwise gone unnoticed.
The effectiveness of causal chambers in producing reliable and real-world applicable data is evident in their application to various AI fields. For instance, they have been used to refine AI approaches like out-of-distribution generalization, change point detection, and independent component analysis. In one example, causal chambers were used to simulate scenarios and test algorithms that predict changes in light intensity based on various sensor inputs and settings.
The innovation of causal chambers represents a significant step forward in addressing the challenge of a lack of real-world datasets for method validation in AI development. These controlled environments provide a unique opportunity to generate diverse and realistic datasets that bridge the gap between theoretical models and practical applications. By allowing researchers to validate AI methodologies in a controlled environment, causal chambers enhance the robustness and applicability of AI models.
In conclusion, the development of causal chambers offers new possibilities for enhancing AI validation and bridging data gaps in machine learning and statistics. These controlled environments simulate physical phenomena, generating diverse datasets that facilitate the validation of AI models in areas with limited access to real-world data. With successful applications across various AI domains, including causal discovery and symbolic regression, causal chambers represent a groundbreaking solution for addressing the lack of real-world datasets in AI research.
Check out the Paper All credit for this research goes to the researchers of this project. Also, don’t forget to follow us on LinkedIn. Do join our active AI community on Discord.
If you like our work, you will love our Newsletter 📰