The Relationship between Compression and Intelligence: Insights from AI Research in China
In recent years, the field of artificial intelligence (AI) has witnessed significant advancements, particularly in the area of language modeling. Language models, such as GPT-3 by OpenAI, have demonstrated impressive capabilities in various natural language processing tasks, from text generation to translation.
However, a fundamental question remains unanswered: what is the relationship between compression and intelligence in AI systems?
Compression, in the context of AI, refers to the ability of a language model to represent information in a more compact form without losing its meaning or utility. Intelligence, on the other hand, encompasses a range of cognitive abilities, including problem-solving, knowledge-understanding, and reasoning. Understanding the relationship between compression and intelligence can provide valuable insights into the core mechanisms of AI systems and potentially push the boundaries of AI development even further.
The Compression-Intelligence Hypothesis
The idea that compression and intelligence are closely related has been a subject of theoretical debate among AI researchers. Many argue that compression is an essential aspect of intelligence, as it enables efficient information processing and storage. According to this hypothesis, an AI system that can compress information effectively is considered more intelligent than a system that cannot.
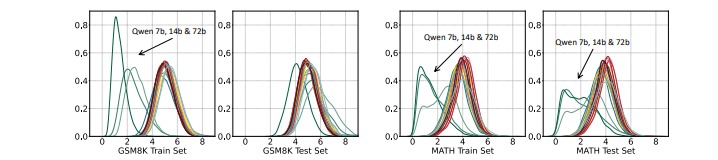
Recent research from China has sought to provide empirical evidence on the relationship between compression and intelligence. The study, conducted by Tencent and The Hong Kong University of Science and Technology, takes a pragmatic approach to intelligence, focusing on the model’s ability to perform downstream tasks rather than delving into philosophical debates about the nature of intelligence.
Empirical Analysis and Findings
To test the compression-intelligence hypothesis, the researchers evaluated several language models’ compression efficiency and their effectiveness in performing various downstream tasks. The study used 30 public language models and 12 different benchmarks to assess the models’ intelligence in specific domains, such as coding skills.
The results of the research were striking, demonstrating a linear relationship between the compression efficiency of language models and their downstream abilities. The study found a Pearson correlation coefficient of approximately -0.95, indicating that as compression efficiency increases, the models’ intelligence, as measured by their performance in downstream tasks, also improves. This linear correlation held true for most individual benchmarks within the study.
Moreover, the study revealed that compression efficiency remained consistent across different model sizes, tokenizers, context window durations, and pre-training data distributions. These findings support the notion that compression efficiency is a reliable metric for assessing language models’ intelligence.
Implications and Future Directions
The empirical evidence provided by the Chinese AI research team offers valuable insights into the relationship between compression and intelligence. The findings suggest that compression efficiency can serve as an unsupervised parameter for language models, enabling easy updating of text corpora and preventing overfitting and test contamination. Researchers can assess language models’ capabilities more effectively by leveraging compression efficiency as a stable and versatile metric.
However, the study acknowledges several limitations and raises intriguing questions for further investigation. The researchers highlight the need to explore the connections between the compression efficiency of base models and the benchmark scores of related improved models. They also suggest studying whether the relationship between compression and intelligence holds true for partially trained or fine-tuned models.
Future research in this area could delve deeper into these questions, expanding the understanding of the connection between compression and intelligence. This line of inquiry holds promise for advancing AI systems’ capabilities and promoting breakthroughs in natural language processing, text generation, and other language-driven domains.
Conclusion
The AI research from China provides empirical evidence that supports the compression-intelligence hypothesis. The study demonstrates a linear relationship between compression efficiency and the downstream abilities of language models, indicating that higher-quality compression corresponds to higher intelligence. The findings have significant implications for the assessment and development of AI systems, particularly in the field of language modeling.
As AI continues to advance, understanding the core principles underlying its intelligence becomes increasingly critical. The relationship between compression and intelligence offers valuable insights into the fundamental mechanisms of AI systems, enabling researchers to push the boundaries of AI development further.
In conclusion, the research from China contributes to our understanding of the intricate relationship between compression and intelligence in AI. It opens up exciting avenues for further research and encourages the AI community to explore the potential implications of compression in advancing AI systems’ capabilities.
Check out the Paper All credit for this research goes to the researchers of this project. Also, don’t forget to follow us on LinkedIn. Do join our active AI community on Discord.
If you like our work, you will love our Newsletter 📰