Google AI Presents Lumiere: A Space-Time Diffusion Model for Video Generation
In recent years, generative models have made significant advancements in tasks such as text-to-image (T2I) generation, producing high-resolution, realistic images from textual prompts. However, extending this capability to text-to-video (T2V) models poses unique challenges due to the complexities introduced by motion. The limitations in existing T2V models include video duration, visual quality, and realistic motion generation. To address these challenges, researchers from Google Research, Weizmann Institute, Tel-Aviv University, and Technion have introduced Lumiere, a groundbreaking text-to-video diffusion model that aims to synthesize videos with realistic, diverse, and coherent motion.
The Challenges of Text-to-Video Generation
While state-of-the-art T2I diffusion models excel at synthesizing high-resolution, photo-realistic images, extending these advancements to T2V models faces challenges due to the complexities of motion. Existing T2V models often employ a cascaded design, where a base model generates keyframes and subsequent temporal super-resolution (TSR) models fill in the gaps. However, limitations in motion coherence persist in these models.
🔥Explore 3500+ AI Tools and 2000+ GPTs at AI Toolhouse
Introducing Lumiere: A Space-Time Diffusion Model
Lumiere introduces a novel approach to text-to-video generation by addressing the challenges of motion synthesis. The model incorporates a Space-Time U-Net architecture, which generates the entire temporal duration of a video in a single pass. This contrasts with existing models that synthesize distant keyframes followed by temporal super-resolution. By leveraging a pre-trained text-to-image diffusion model and incorporating spatial and temporal down- and up-sampling, Lumiere achieves state-of-the-art text-to-video results.
Efficient Processing with Space-Time U-Net
Lumiere efficiently processes both spatial and temporal dimensions using its Space-Time U-Net architecture. The model generates full video clips at a coarse resolution, utilizing temporal blocks with factorized space-time convolutions and attention mechanisms for effective computation. By leveraging a pre-trained text-to-image architecture, Lumiere maintains coherence and ensures smooth transitions between temporal segments. The introduction of multidiffusion enables spatial super-resolution, addressing memory constraints.
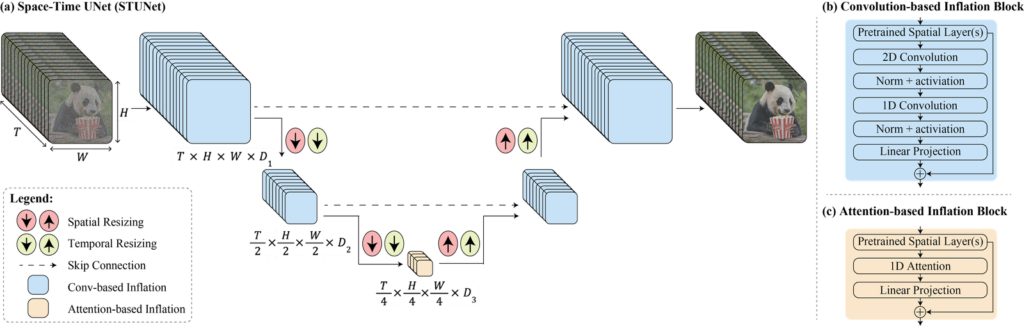
Outperforming Existing Models
Lumiere surpasses existing models in video synthesis. Trained on a dataset of 30 million 80-frame videos, Lumiere outperforms models such as ImagenVideo, AnimateDiff, and ZeroScope in qualitative and quantitative evaluations. The model demonstrates superior motion coherence by achieving competitive Frechet Video Distance and Inception Score in zero-shot testing on UCF101. User studies confirm Lumiere’s preference over various baselines, including commercial models, highlighting its excellence in visual quality and alignment with text prompts.
Versatility and Applications
Lumiere’s state-of-the-art results highlight the versatility of the approach for various applications. In addition to text-to-video generation, the model can be applied to image-to-video synthesis, video inpainting, and stylized generation. The efficient processing capabilities of Lumiere make it suitable for content creation and video editing tasks.
Conclusion
The introduction of Lumiere, a text-to-video diffusion model, represents a significant advancement in the field of video generation. By addressing the challenges of motion synthesis and incorporating a Space-Time U-Net architecture, Lumiere achieves state-of-the-art results in terms of visual quality, motion coherence, and alignment with text prompts. The model’s efficient processing capabilities and versatility make it a valuable tool for content creators, video editors, and researchers in the field of generative models.
Overall, Lumiere marks a pivotal moment in the development of text-to-video generation models, paving the way for realistic, diverse, and coherent motion synthesis in video generation.
Check out the Paper and Project Page. All credit for this research goes to the researchers of this project. Also, don’t forget to follow us on LinkedIn. Do join our active AI community on Discord.
If you like our work, you will love our Newsletter 📰