Boost AI Clarity with T-Explainer: A New Tool
In today’s world, machine learning models are being employed in various sectors, including healthcare, finance, and other critical applications. However, as these models grow in complexity, their lack of transparency becomes a significant concern. The decision-making process of these models often remains a mystery, leading to a lack of trust and accountability.
To address this issue, researchers from the University of São Paulo (ICMC-USP), New York University (NYU), and Capital One have introduced a novel AI framework called T-Explainer. This framework aims to provide consistent and reliable explanations for machine learning models, enhancing trust and facilitating a more informed decision-making process.
The Challenge of Transparency in Complex Machine Learning Models
As machine learning models become more complex, they tend to resemble “black boxes” where the reasoning behind their predictions is opaque. In domains such as healthcare and finance, understanding the basis of decisions is crucial. However, traditional methods of increasing model transparency, such as feature attribution techniques, often suffer from inconsistencies. These methods can provide different explanations for the same input under various conditions, undermining their reliability and trustworthiness.
Introducing T-Explainer: A Framework for Transparent Machine Learning Models
The T-Explainer framework addresses the challenge of inconsistent explanations by leveraging local additive explanations based on Taylor expansions, a robust mathematical principle. Unlike other methods that may yield fluctuating outputs, T-Explainer operates through a deterministic process, ensuring stability and repeatability in its results.
The framework aims to identify the features of a machine learning model that influence its predictions, providing precise and insightful explanations. Through a series of benchmark tests, T-Explainer has demonstrated its superiority over established methods like SHAP and LIME in terms of stability and reliability. It consistently maintains explanation accuracy across multiple assessments, outperforming other frameworks in stability metrics such as Relative Input Stability (RIS) and Relative Output Stability (ROS).
Advantages of T-Explainer: Stability, Reliability, and Flexibility
One of the key advantages of T-Explainer is its stability and reliability in providing explanations. Unlike gradient-based attribution methods that can yield divergent explanations for the same input, T-Explainer ensures consistency in its results. This stability is crucial for building trust in AI systems and facilitating more informed decision-making.
Another important aspect of T-Explainer is its flexibility and ability to integrate seamlessly with existing frameworks. It can be applied effectively across various types of machine learning models, making it a versatile tool for explanation generation. This flexibility sets T-Explainer apart from other explanatory frameworks, which may not offer the same level of adaptability.
Benchmark Tests and Comparative Evaluations
To demonstrate the effectiveness of T-Explainer, researchers conducted benchmark tests and comparative evaluations against established methods like SHAP and LIME. In these evaluations, T-Explainer consistently outperformed other frameworks in terms of stability and reliability metrics.
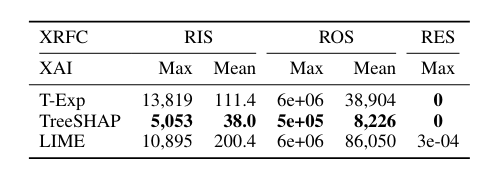
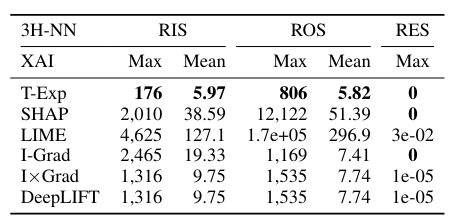
For example, in terms of Relative Input Stability (RIS) and Relative Output Stability (ROS), T-Explainer demonstrated superior performance. These metrics assess the stability of explanations by measuring how much the explanations change with slight variations in the input or output. T-Explainer’s ability to maintain stability in these metrics showcases its reliability as an explanatory framework.
The Impact of T-Explainer on AI Applications
By providing consistent and reliable explanations, T-Explainer addresses the critical need for transparency in machine learning models. The framework enhances the trust in AI systems and enables a more informed decision-making process, particularly in domains where accountability is paramount.
The impact of T-Explainer extends beyond individual models. Its ability to integrate with existing frameworks and its flexibility make it a valuable tool for improving the transparency and trustworthiness of AI applications as a whole. With T-Explainer, AI systems can become more accountable and interpretable, setting a new standard for explainability.
Conclusion
Transparency in machine learning models is a fundamental requirement for their adoption and trustworthiness. The introduction of T-Explainer by researchers from ICMC-USP, NYU, and Capital One addresses the challenge of inconsistent and unreliable model explanations. By leveraging Taylor expansions and operating through a deterministic process, T-Explainer provides stable, reliable, and precise explanations for machine learning models.
Through benchmark tests and comparative evaluations, T-Explainer has demonstrated its superiority over established methods, showcasing its impact on the transparency and trustworthiness of AI applications. The framework’s ability to seamlessly integrate with existing frameworks and its flexibility make it an invaluable tool in critical applications where decision-making must be informed and accountable.
T-Explainer sets a new standard for consistent and reliable machine learning model explanations, paving the way for a more accountable and interpretable future in AI systems.
Check out the Paper. All credit for this research goes to the researchers of this project. Also, don’t forget to follow us on LinkedIn. Do join our active AI community on Discord.
Explore 3600+ latest AI tools at AI Toolhouse 🚀.
Read our other blogs on AI Tools 😁
If you like our work, you will love our Newsletter 📰