MAmmoTH2 and MAmmoTH2-Plus: Boosting AI Capabilities with Web-Instruct
Large language models (LLMs) have revolutionized natural language processing by enabling machines to understand and generate human-like text. These models have shown remarkable performance across various tasks such as language translation, sentiment analysis, and question answering. However, to further enhance their reasoning abilities and broaden their application scope, instruction tuning plays a crucial role.
Instruction tuning involves fine-tuning the large language models with high-quality instruction data to improve their ability to solve new, unseen problems effectively. Traditionally, acquiring such data has been a challenging task due to high costs, limited scalability, and potential biases. However, a groundbreaking approach called Web-Instruct has emerged, harnessing the power of web-mined data to address these limitations and enhance large language models like MAmmoTH2 and MAmmoTH2-Plus.
The Need for Instruction Tuning in Large Language Models
Large language models are trained on vast amounts of text data to learn patterns, relationships, and contextual understanding. However, training these models solely on raw text data may not be sufficient to optimize their performance in specific tasks. Instruction tuning aims to bridge this gap by fine-tuning the models with targeted instruction data that helps them reason and solve complex problems effectively.
The traditional methods of acquiring instruction data often involve human input or complex algorithms to distill complex datasets into usable training materials. These methods are costly, time-consuming, and may introduce biases. Hence, there is a need for a more efficient and scalable approach to acquire high-quality instruction data.
Web-Instruct: Leveraging Web-Mined Data for Instruction Tuning
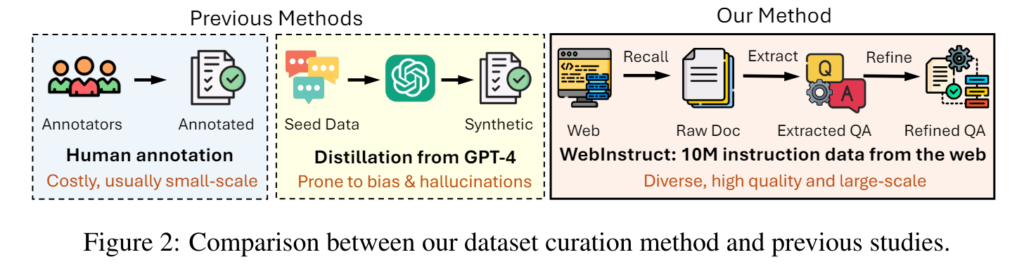
Web-Instruct is an innovative approach developed by researchers from Carnegie Mellon University and the University of Waterloo. It harnesses the power of web-mined data to acquire large-scale instruction datasets for fine-tuning large language models like MAmmoTH2 and MAmmoTH2-Plus.
The Web-Instruct method involves several steps to extract relevant instruction-response pairs from the vast online content and refine them for high quality and relevance. Here’s an overview of the process:
- Web Corpus Selection: A broad web corpus is selected, comprising diverse online documents such as articles, blogs, forums, and instructional websites. This ensures a wide range of instructional content from various domains.
- Instruction-Response Pair Extraction: From the selected web corpus, potential instruction-response pairs are extracted using natural language processing techniques. These pairs capture the instructions and the corresponding responses or solutions present in the web content.
- Quality and Relevance Refinement: The extracted instruction-response pairs undergo a refinement process to filter out noise, eliminate irrelevant or low-quality pairs, and ensure the dataset’s overall high quality. This step is crucial to ensure that the fine-tuned models receive accurate and reliable instruction data.
By leveraging web-mined data, the Web-Instruct approach overcomes the limitations of traditional instruction data acquisition methods. It enables the acquisition of massive and diverse datasets without relying on costly human data curation or biased model distillation methods.
MAmmoTH2 and MAmmoTH2-Plus: The Power of Web-Mined Instruction Data
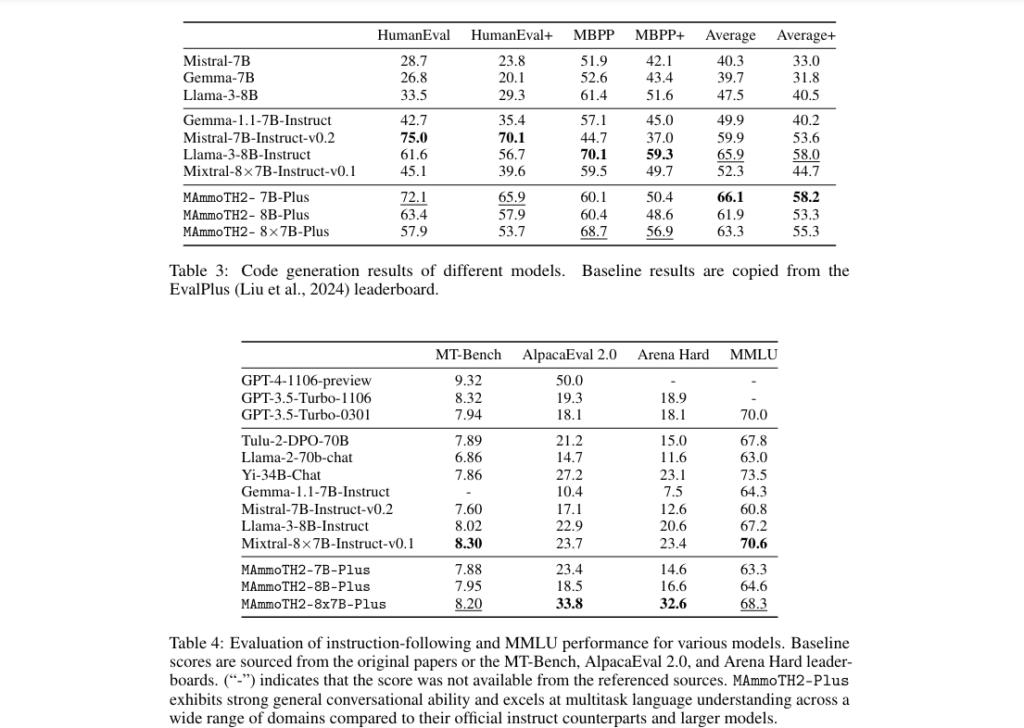
The instruction tuning process using Web-Instruct has resulted in the development of two powerful large language models: MAmmoTH2 and MAmmoTH2-Plus. These models showcase the effectiveness of web-mined instruction data in enhancing the reasoning capabilities of LLMs.
MAmmoTH2
MAmmoTH2 is a large language model fine-tuned using the Web-Instruct dataset, which comprises approximately 10 million high-quality instruction-response pairs. This dataset, acquired through the Web-Instruct method, eliminates the need for expensive human data curation and mitigates biases introduced through other data collection methods.
The fine-tuning of MAmmoTH2 with the Web-Instruct dataset has yielded remarkable performance improvements. In complex reasoning tasks, such as mathematical problem-solving and scientific reasoning, MAmmoTH2 has demonstrated a significant surge in accuracy from 11% to 34%. These improvements highlight the power of web-mined instruction data in enhancing the reasoning abilities of large language models.
MAmmoTH2-Plus
Building upon the success of MAmmoTH2, researchers have developed an enhanced model called MAmmoTH2-Plus. This variant integrates additional public instruction datasets, further broadening the training scope and enhancing the model’s generalization capabilities.
MAmmoTH2-Plus has consistently outperformed base models on standard reasoning benchmarks like TheoremQA and GSM8K, showcasing its superior performance. It has achieved performance improvements of up to 23% compared to previous benchmarks, indicating its enhanced reasoning abilities across a spectrum of complex reasoning and conversational tasks.
The Advantages of Web-Mined Instruction Data
The utilization of web-mined instruction data through the Web-Instruct method offers several advantages in instruction tuning for large language models:
- Scalability: Web-mined instruction data allows for the acquisition of massive datasets without the need for expensive human data curation. This scalability enables training large language models on diverse and extensive instruction data, enhancing their performance across various domains.
- Cost-effectiveness: Traditional methods of acquiring high-quality instruction data often involve significant costs. Web-mined instruction data, on the other hand, can be acquired at a fraction of the cost, making it a cost-effective alternative.
- Diversity: The web is a vast repository of diverse content, covering a wide range of topics and domains. Leveraging web-mined data ensures that large language models are exposed to a diverse set of instructions and responses, improving their generalization capabilities.
- Real-world relevance: Web-mined instruction data reflects real-world scenarios and challenges, making it more relevant for instruction tuning. This real-world relevance helps large language models understand and solve problems in practical applications.
The success of models like MAmmoTH2 and MAmmoTH2-Plus, fine-tuned with web-mined instruction data, further emphasizes the potential of this approach in enhancing the reasoning abilities of large language models.
Conclusion
Web-Instruct’s instruction tuning approach, leveraging web-mined instruction data, has revolutionized the process of enhancing large language models like MAmmoTH2 and MAmmoTH2-Plus. By acquiring massive and diverse instruction datasets without the constraints of cost and bias, this approach has significantly improved the reasoning capabilities of these models.
The utilization of web-mined instruction data offers scalability, cost-effectiveness, diversity, and real-world relevance in instruction tuning. These advantages open new possibilities for large language models to excel in various domains and tackle complex reasoning tasks.
As the field of instruction tuning continues to advance, the power of web-mined data will play a pivotal role in enhancing the performance of large language models, setting new benchmarks for data quality and model capabilities in the field of AI.
Check out the Paper. All credit for this research goes to the researchers of this project. Also, don’t forget to follow us on LinkedIn. Do join our active AI community on Discord.
Explore 3600+ latest AI tools at AI Toolhouse 🚀.
Read our other blogs on AI Tools 😁
If you like our work, you will love our Newsletter 📰