The Urgent Need for Standardized Data Provenance Frameworks in Enhancing AI Integrity
Artificial intelligence (AI) has revolutionized various industries, ranging from healthcare to finance, by augmenting human capabilities and automating complex tasks. However, the rapid advancement of AI technology also brings forth challenges that need to be addressed. One of the critical issues facing AI development is the need for standardized data provenance frameworks to enhance AI integrity.
The Role of Data in AI Development
AI systems rely on vast datasets for training and learning. These datasets, often sourced from diverse internet resources such as social media, news outlets, and public databases, provide the foundation for AI algorithms. However, the integrity and quality of the data used can significantly impact the performance and ethical implications of AI models.
Challenges in Maintaining Data Integrity and Ethical Standards
The training of AI models, particularly generative models like GPT-4, Gemini, and Cluade, heavily relies on large-scale datasets. Unfortunately, many of these datasets lack proper documentation and vetting, making it challenging to ensure data integrity and ethical standards. This lack of transparency poses risks such as privacy violations and the perpetuation of biases within AI systems.
Fragmented Data Provenance Practices
Existing tools and methods for tracking data provenance in AI are often fragmented and inadequate in addressing the complexity of diverse data sources. Many of these tools focus on specific aspects of data management and overlook interoperability with other data governance frameworks. Consequently, there is a pressing need for a unified system that comprehensively addresses transparency, authenticity, and consent in AI data.
The Proposed Standardized Data Provenance Framework
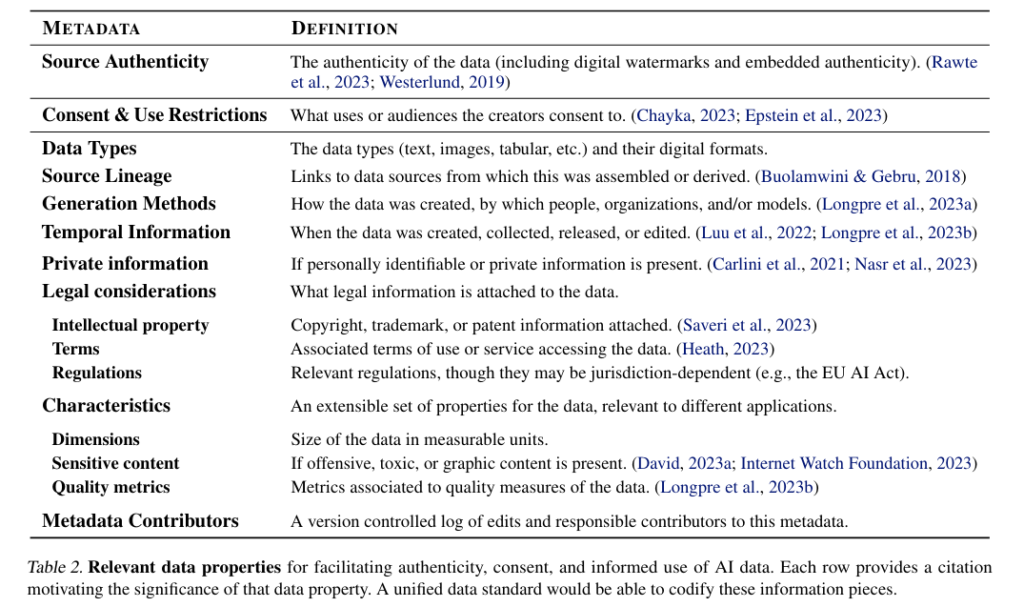
To tackle the urgent need for standardized data provenance frameworks, researchers from MIT and Harvard University have proposed a comprehensive framework for data management in AI. This framework aims to ensure transparency, authenticity, and consent by requiring detailed documentation of data sources and permissions.
Metadata Documentation
The proposed framework emphasizes the establishment of a searchable, structured library that logs metadata concerning the origin and usage permissions of data. This documentation would enable AI developers to access and utilize data responsibly while being aware of its origins and usage restrictions.
Transparent Environment
By fostering a transparent environment, the proposed framework promotes ethical AI development. Developers can make informed choices about the datasets they use, mitigating legal risks and reducing incidents of non-consensual data usage or copyright disputes. Transparently sourced data can also enhance the reliability of AI models by reducing privacy breaches and biases.
Legal Implications
The implementation of robust data provenance practices can have significant legal implications. Recent industry cases have shown that companies using transparently sourced data are less likely to face litigation related to data misuse. Analysis suggests that the adoption of comprehensive data provenance frameworks could lead to a potential decrease of up to 40% in legal actions.
Benefits of a Standardized Data Provenance Framework
The establishment of a standardized data provenance framework offers several benefits for AI development and society as a whole.
Ethical AI Development
By ensuring transparency, authenticity, and consent in data usage, a standardized framework promotes ethical AI development. It allows AI developers to make informed decisions about the data they use, reducing the risk of perpetuating biases or violating privacy rights.
Enhanced Data Integrity
With a comprehensive data provenance framework, developers can confidently rely on well-documented and ethically sourced data, improving the integrity of AI models. This, in turn, leads to more reliable and trustworthy AI technologies.
Legal Risk Mitigation
Standardized data provenance frameworks can help mitigate legal risks associated with AI development. Companies that adhere to rigorous data provenance practices are less likely to face litigation related to data misuse, protecting both their reputation and financial stability.
Public Trust and Acceptance
The establishment of standardized data provenance frameworks fosters public trust in AI applications. Concerns surrounding privacy violations and biases are addressed through transparent data usage. As a result, AI technologies are more likely to gain societal acceptance and support.
Conclusion
The urgent need for standardized data provenance frameworks in enhancing AI integrity is a critical challenge for the AI community. Researchers from MIT and Harvard University have proposed a comprehensive framework that addresses the issues of transparency, authenticity, and consent in AI data usage. By implementing such a framework, AI developers can make informed decisions, mitigate legal risks, and foster public trust in AI technologies.
As AI continues to shape various industries, the development of standardized data provenance frameworks becomes increasingly crucial. It is essential to prioritize the ethical and transparent use of data to ensure the responsible advancement of AI and its positive impact on society.
Check out the Paper. All credit for this research goes to the researchers of this project. Also, don’t forget to follow us on LinkedIn. Do join our active AI community on Discord.Explore 3600+ latest AI tools at AI Toolhouse 🚀.
Read our other blogs on AI Tools 😁
If you like our work, you will love our Newsletter 📰