Network Optimization with AI: Exploring Predictive Maintenance and Traffic Management
In today’s digital age, the efficiency and reliability of networks, whether they are telecommunications frameworks or urban traffic systems, are pivotal. Artificial Intelligence (AI) is crucial in enhancing these networks through predictive maintenance and advanced traffic management techniques. This article explores how these AI-driven methodologies are transforming the landscape of network optimization.
Predictive Maintenance: Enhancing Network Efficiency
Predictive maintenance refers to predicting when an equipment failure might occur and conducting maintenance to prevent it. Traditionally, maintenance activities are performed based on fixed schedules or reactive responses to failures. However, these approaches are often inefficient and costly.
Explore 3600+ latest AI tools at AI Toolhouse 🚀
By utilizing AI algorithms and machine learning techniques, predictive maintenance brings a proactive approach to network optimization. It leverages historical data, real-time sensor data, and advanced analytics to identify patterns and anomalies. With these insights, maintenance activities can be planned in advance, minimizing downtime and optimizing resource allocation.
Key Elements of Predictive Maintenance:
- Data collection: Extensive data collection from various sources, such as sensors, logs, and historical records, enables the training of AI models.
- Data analysis: AI algorithms analyze the collected data to identify patterns and detect early signs of equipment failure.
- Predictive modeling: Through machine learning techniques, predictive models are built to forecast when maintenance actions should be taken.
- Optimization of maintenance activities: By prioritizing maintenance tasks based on predicted failures, resources can be allocated efficiently, reducing costs and minimizing disruptions.
Benefits of Predictive Maintenance:
Predictive maintenance offers several advantages over traditional maintenance approaches:
- Reduced downtime: By identifying and addressing potential failures in advance, network downtime can be minimized, ensuring high uptime and uninterrupted services.
- Cost savings: Proactive maintenance reduces the likelihood of costly emergency repairs and optimizes the utilization of resources, resulting in significant cost savings.
- Increased equipment lifespan: Regular monitoring and timely maintenance prevent equipment degradation, extending their lifespan and avoiding premature replacements.
- Improved safety: Predictive maintenance ensures a safer network environment by identifying and addressing potential safety hazards before they cause accidents.
AI-driven Traffic Management: Optimizing Urban Traffic Systems
In addition to predictive maintenance, AI is playing a transformative role in traffic management. AI-driven traffic management systems analyze real-time data, such as vehicle flow, speed, and congestion, to optimize traffic flow, reduce congestion, and enhance road safety. These systems leverage AI algorithms and machine learning techniques to make data-driven decisions and dynamically adjust traffic signals and route guidance.
Components of AI-based Traffic Management:
- Traffic Data Collection: Sensors, cameras, and other data collection devices populate the traffic management system with real-time information about traffic conditions.
- Data Analytics: AI algorithms process the collected data to identify traffic patterns, congestion hotspots, and potential bottlenecks.
- Route Optimization: Using the analyzed data, AI-based systems optimize traffic routes, considering factors like travel time, traffic volume, and road conditions.
- Adaptive Traffic Signal Control: AI algorithms dynamically adjust traffic signal timings based on real-time traffic data, ensuring optimized traffic flow and reducing congestion.
Key Applications of AI-based Traffic Management:
- Congestion Management: AI-driven traffic management systems identify congested areas and suggest alternate routes to redirect traffic flow and alleviate congestion.
- Accident Prevention: Real-time analysis of traffic data helps identify potential accident-prone areas. AI algorithms can employ predictive analytics to proactively address these locations and prevent accidents.
- Public Transportation Optimization: AI-based systems optimize public transportation routes and schedules to ensure efficient services and reduce waiting times.
- Emergency Vehicle Management: Traffic management systems can prioritize emergency vehicles’ passage by dynamically adjusting traffic signal timings and clearing the route.
Integrating predictive maintenance and AI-driven traffic management can substantially improve public transportation and urban traffic systems. By synchronizing maintenance activities with traffic optimization strategies, disruptions can be minimized, and overall network efficiency can be maximized.
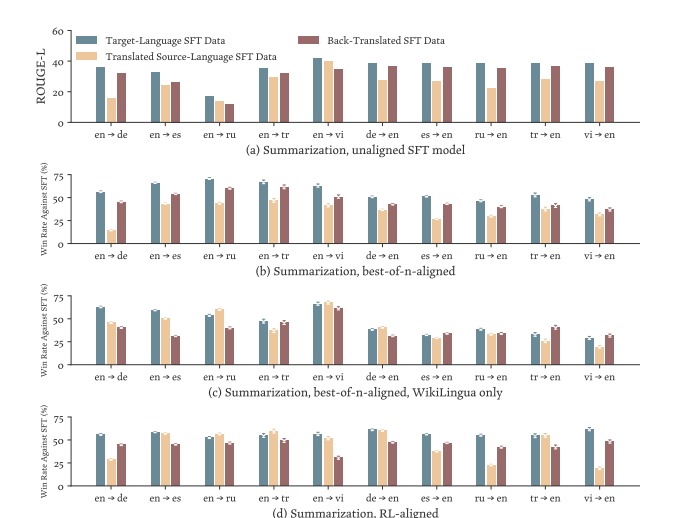
Overcoming Challenges and Future Prospects:
Despite the clear benefits, integrating AI in network optimization faces several challenges:
Challenges:
- Privacy concerns: Effective network optimization requires access to a vast amount of data, which raises concerns about privacy and data security. Careful handling of sensitive information is necessary to build trust among network users and stakeholders.
- Technical complexity: Implementing AI-driven network optimization solutions requires advanced technical expertise and infrastructure. Organizations need to invest in skilled personnel, AI tools, and robust network infrastructure to fully realize the potential of AI in network optimization.
- Infrastructure adaptation: Legacy networks and systems may not be compatible with AI-driven optimization solutions, requiring significant modifications or upgrades.
Future Prospects:
The future of network optimization with AI looks promising. Advancements in AI technologies, such as natural language processing and computer vision, can further enhance predictive maintenance and traffic management capabilities. Additionally, the increasing availability of real-time data from sensors, IoT devices, and connected vehicles will provide a rich source of information for AI algorithms to make more accurate predictions and optimizations.
Through predictive maintenance and traffic management, AI’s role in network optimization transforms networks’ operations. It enhances efficiency, reduces costs, and improves service quality. However, realizing its full potential requires overcoming significant challenges related to privacy, technical complexity, and infrastructure adaptation. By addressing these challenges, we can pave the way for smarter, more reliable networks that significantly benefit both businesses and consumers.
In conclusion, AI-driven optimization strategies are not just about maintaining the status quo but are key to future-proofing network infrastructures against an increasingly dynamic and demanding future. The combination of predictive maintenance and traffic management holds immense potential for revolutionizing network optimization and delivering seamless experiences for users and communities alike.
Check out the Paper. All credit for this research goes to the researchers of this project. Also, don’t forget to follow us on LinkedIn. Do join our active AI community on Discord.
If you like our work, you will love our Newsletter 📰