Advancing Vision-Language Models: Overcoming Hallucination Challenges
The field of artificial intelligence has witnessed significant advancements in recent years, particularly in the area of Vision-Language Models (VLMs). These models, which combine visual perception and language processing, offer great potential for enabling machines to understand and describe the world around us. However, one major challenge that researchers face is the issue of hallucination, where the generated text does not accurately reflect the visual input. In this article, we will explore the efforts made by Huawei Technologies researchers to overcome these hallucination challenges and advance the field of Vision-Language Models.
Understanding Hallucination Challenges in Vision-Language Models
Before delving into the solutions proposed by Huawei Technologies researchers, it is important to understand the root causes of hallucination challenges in Vision-Language Models. Hallucination occurs when there is a disconnect between the visual data and the text generated by the model. This can arise due to limitations in the model’s design and training data, which may introduce biases or hinder the model’s ability to fully comprehend the visual information 1. Such hallucinations can undermine the reliability and accuracy of VLMs in critical applications.
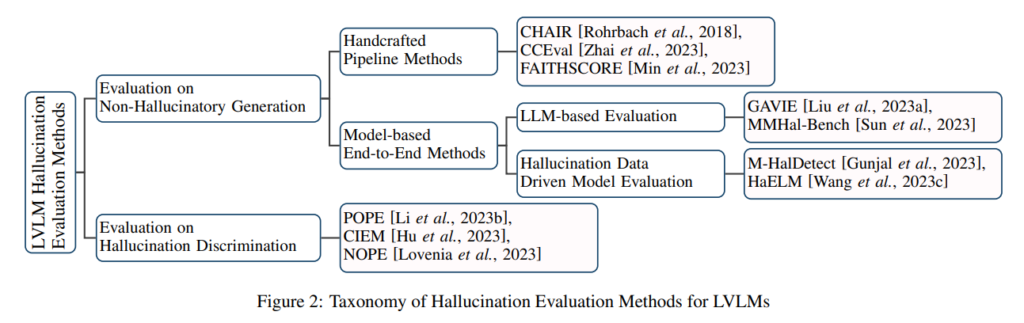
Exploring Innovative Strategies to Overcome Hallucination Challenges
The researchers at Huawei Technologies have taken a systematic approach to address hallucination challenges in Vision-Language Models. They have proposed several innovative strategies to refine the core components of these models, ultimately reducing the occurrence of hallucinatory outputs.
Enhancing Data Processing Techniques
One of the key strategies proposed by the researchers is to develop advanced data processing techniques that improve the quality and relevance of training data 1. By ensuring that the training data represents a diverse range of visual inputs, the models can learn to produce more accurate and contextually appropriate textual descriptions. This approach helps to mitigate the hallucination problem by providing a solid foundation for the models’ learning processes.
Optimizing Visual Encoders and Modality Alignment Mechanisms
Another important aspect that the researchers focus on is the optimization of visual encoders and modality alignment mechanisms. These improvements enable the models to more effectively integrate and process visual and textual information, thereby reducing the likelihood of hallucinatory outputs 1. By fine-tuning the architecture of VLMs, the researchers at Huawei Technologies aim to enhance the models’ ability to capture and represent the semantics of visual data accurately.
Evaluating Performance and Identifying Key Factors
To evaluate the performance of Vision-Language Models and identify factors contributing to hallucination, the researchers employ a comprehensive set of benchmarks 1. These benchmarks measure the prevalence of hallucinations in the model’s output and provide insights into the quality of visual encoders, the effectiveness of modality alignment, and the models’ ability to maintain context awareness during the generation process. This evaluation allows the researchers to pinpoint specific areas for improvement and develop targeted interventions.
Marked Improvement in Accuracy and Reliability
The efforts made by Huawei Technologies researchers have yielded promising results. After implementing the proposed solutions, the researchers report a marked improvement in the accuracy and reliability of the generated text. The models now demonstrate an enhanced ability to produce descriptions that closely mirror the factual content of images, thereby reducing instances of hallucination. These results signify the potential of Vision-Language Models to transform various sectors, including automated content creation and assistive technologies.
Looking Ahead: The Future of Vision-Language Models
While the research conducted by Huawei Technologies researchers represents a significant step forward in addressing hallucination challenges, there is still much to be explored in the field of Vision-Language Models. The study concludes by emphasizing the importance of continued innovation in data processing, model architecture, and training methodologies to fully unlock the potential of these models. By refining the core components of Vision-Language Models and addressing the issue of hallucination, researchers aim to create machines with a deep, human-like understanding of visual and textual data.
Conclusion
Advancing Vision-Language Models and overcoming hallucination challenges is a critical area of research in the field of artificial intelligence. The work done by Huawei Technologies researchers highlights the potential of these models to enable machines to understand and describe the visual world with greater accuracy and reliability. By employing innovative strategies such as enhancing data processing techniques and optimizing model architecture, researchers are making significant progress in reducing hallucination instances in Vision-Language Models. As this research continues to evolve, we can expect further advancements that will revolutionize how machines interact with the visual environment, opening up new possibilities for human-machine interactions and applications across various industries.
Check out the Paper. All credit for this research goes to the researchers of this project. Also, don’t forget to follow us on LinkedIn. Do join our active AI community on Discord.
If you like our work, you will love our Newsletter 📰