ByteDance Proposes Magic-Me: A New AI Framework for Video Generation with Customized Identity
In recent years, the field of generative models has seen significant advancements in text-to-image (T2I) and text-to-video (T2V) generation. These advancements have opened up new possibilities for creating custom content and have led to the development of innovative frameworks and models. One such framework is Magic-Me, proposed by researchers from ByteDance Inc. and UC Berkeley. Magic-Me is an AI framework specifically designed for video generation with customized identity. In this article, we will explore the key features and advancements of Magic-Me and its implications for the future of video generation.
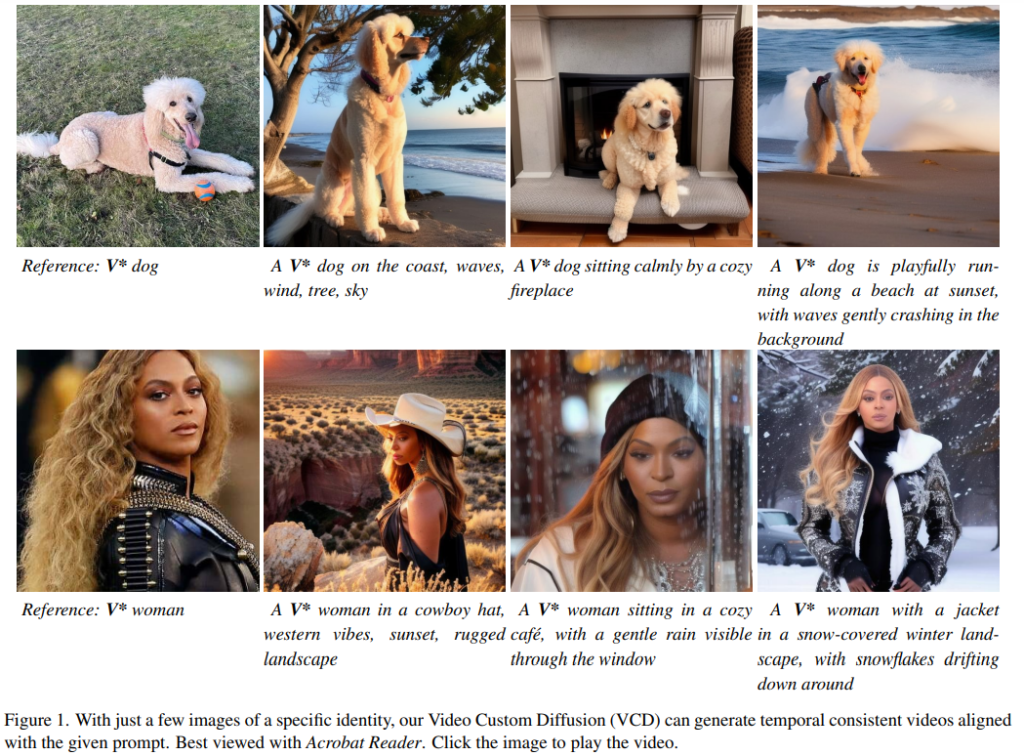
The Challenge of Customized Identity in Video Generation
While T2I models have made great strides in controlling subject identity, extending this capability to T2V generation has proven to be more challenging. Existing T2V methods struggle to maintain consistent identities and stable backgrounds across frames. This is primarily due to the influence of diverse reference images on identity tokens and the difficulty of ensuring temporal consistency in the presence of varying identity inputs.
Introducing Magic-Me: A Framework for Customized Identity in Video Generation
Researchers from ByteDance Inc. and UC Berkeley have developed Video Custom Diffusion (VCD), a powerful and straightforward framework for generating subject identity-controllable videos. VCD employs three key components that address the challenges mentioned earlier:
- ID Module: The ID module is responsible for precise identity extraction. By disentangling identity information from background noise, the ID module ensures accurate alignment of identity tokens, resulting in stable video outputs.
- 3D Gaussian Noise Prior: To maintain inter-frame consistency, VCD utilizes a 3D Gaussian Noise Prior. This component helps mitigate exposure bias during inference, enhancing the overall quality of the generated videos.
- V2V Modules: VCD incorporates V2V (video-to-video) modules to enhance video quality. These modules contribute to robust denoising techniques, resolution enhancement, and a training approach for noise mitigation in identity tokens.
Advancements in Identity-Specific Video Generation
With the development of T2I generation techniques, customizable models capable of creating realistic portraits and imaginative compositions have emerged. Techniques like Textual Inversion and DreamBooth have allowed for fine-tuning pre-trained models with subject-specific images, resulting in the generation of unique identifiers linked to desired subjects. This progress has extended to multi-subject generation, where models can compose multiple subjects into single images.
However, transitioning from T2I to T2V generation presents new challenges, primarily the need for spatial and temporal consistency across frames. While early methods utilized techniques such as Generative Adversarial Networks (GANs) and Variational Autoencoders (VAEs) for low-resolution videos, recent approaches, including Magic-Me, have leveraged diffusion models for higher-quality output.
The Components of Magic-Me
Let’s dive deeper into the components that make up the Magic-Me framework:
Preprocessing Module
Magic-Me incorporates a preprocessing module that prepares input data for the subsequent stages of video generation. This module ensures that the input text or reference images are appropriately formatted and processed.
ID Module
The ID module is a crucial component of Magic-Me. It incorporates extended ID tokens with masked loss and prompt-to-segmentation techniques for precise identity disentanglement. By removing background noise, the ID module allows for accurate alignment of identity information across frames.
Motion Module
The motion module in Magic-Me is responsible for maintaining temporal consistency in the generated videos. It ensures smooth transitions between frames, contributing to a seamless viewing experience.
ControlNet Tile Module
The ControlNet Tile module is an optional component that can be used to upsample videos, resulting in higher resolution. This module further enhances the visual quality of the generated videos while preserving identity details.
V2V Pipelines: Face VCD and Tiled VCD
Magic-Me introduces two V2V pipelines: Face VCD and Tiled VCD.
- Face VCD focuses on enhancing facial features and resolution in the generated videos. This pipeline ensures that identity-related details, such as facial expressions and characteristics, are accurately represented.
- Tiled VCD goes a step further by upscaling the video while preserving identity details. This pipeline is particularly useful when generating videos that require higher resolution.
Evaluation and Performance
To evaluate the performance of the Magic-Me framework, the researchers conducted extensive experiments. They selected subjects from diverse datasets and compared the results against multiple baselines using identity alignment metrics such as CLIP-I and DINO, text alignment, and temporal smoothness.
The training details of Magic-Me involved using Stable Diffusion 1.5 for the ID module, with adjustments made to learning rates and batch sizes accordingly. The researchers sourced data from DreamBooth and CustomConcept101 datasets, which allowed them to evaluate the model’s performance against various metrics.
The study highlighted the critical role of the 3D Gaussian Noise Prior and the prompt-to-segmentation module in enhancing video smoothness and image alignment. Realistic Vision, a component of Magic-Me, generally outperformed Stable Diffusion, emphasizing the importance of choosing the appropriate model for video generation.
Implications and Future Directions
The introduction of the Magic-Me framework marks a significant advancement in subject identity-controllable video generation. By seamlessly integrating identity information and frame-wise correlation, Magic-Me sets a new benchmark for video identity preservation.
The adaptability of Magic-Me with existing text-to-image models enhances its practicality and usability in various applications. The availability of features such as the 3D Gaussian Noise Prior and the Face/Tiled VCD modules ensures stability, clarity, and higher resolution in the generated videos.
Further research and development in this field hold great potential for improving the quality and customization capabilities of video generation models. As AI frameworks like Magic-Me continue to evolve, we can expect more realistic and personalized video content in the future.
In conclusion, ByteDance’s proposal of Magic-Me demonstrates the company’s commitment to pushing the boundaries of AI technology in the field of video generation. The framework’s ability to generate videos with customized identities opens up new possibilities for content creators, filmmakers, and digital artists. With its impressive advancements in identity-specific video generation, Magic-Me sets a new standard for high-quality video production. As this technology continues to progress, we can look forward to the emergence of even more sophisticated and personalized video content.
Check out the Paper. All credit for this research goes to the researchers of this project. Also, don’t forget to follow us on LinkedIn. Do join our active AI community on Discord.
If you like our work, you will love our Newsletter 📰