Decoding AI Cognition: Unveiling the Color Perception of Large Language Models through Cognitive Psychology Methods
Artificial intelligence (AI) has made significant strides in recent years, but the inner workings of these complex systems remain a mystery. Understanding how AI models perceive and process information is crucial for further advancements in the field. In a groundbreaking study, researchers have employed cognitive psychology methods to uncover the color perception of Large Language Models (LLMs), shedding light on their conceptualization of color space.
The Challenge of Interpreting AI Models
Interpreting AI models is a challenging task due to their complexity and opaque nature. Previous techniques involved analyzing the activation patterns of artificial neurons, but as models grow more sophisticated, these methods struggle to keep up. To overcome this challenge, researchers have turned to cognitive psychology, borrowing methodologies to gain insights into AI systems’ mental representations.
🔥Explore 3500+ AI Tools and 2000+ GPTs at AI Toolhouse
Unveiling the Color Perception of GPT-4
Researchers from Princeton University and the University of Warwick have delved into the color perception of GPT-4, a prominent Large Language Model. The study focuses on inferring GPT-4’s mental representations through its responses to specific probes, and mirroring techniques used in cognitive psychology to understand human cognition.
Methodology: Direct Sampling and MCMC
To investigate GPT-4’s mental representations, the researchers employed direct sampling and Markov Chain Monte Carlo (MCMC) methods, with a specific focus on color perception. This methodological choice provides a more nuanced and efficient way to understand AI’s thoughts. By simulating scenarios related to color choices or tasks, the study aims to map out GPT-4’s conceptualization of color space, similar to studying human cognition.
A Multifaceted Approach to Unveiling AI’s Color Perception
The study’s methodology encompasses various techniques to probe GPT-4’s perception of color, providing a comprehensive analysis. These techniques include direct prompting, direct sampling, MCMC, and Gibbs sampling. Each approach offers unique insights into GPT-4’s understanding of color.
Direct Prompting
Direct prompting involves asking GPT-4 to generate HSL (Hue, Saturation, Lightness) color codes for given objects. This technique allows researchers to observe how GPT-4 associates colors with specific entities or concepts, providing insights into its mental representations of color.
Direct Sampling
Direct sampling evaluates GPT-4’s binary responses to randomly selected colors. By studying how GPT-4 categorizes colors, researchers can gain a deeper understanding of its color perception. This approach sheds light on how GPT-4 organizes and interprets the vast spectrum of colors.
MCMC and Gibbs Sampling
The researchers utilized adaptive methods like MCMC and Gibbs sampling to iteratively refine GPT-4’s responses. These techniques allow for a dynamic and nuanced exploration of the AI’s color representations. By refining its responses, the researchers can uncover more accurate and human-like color conceptualizations within GPT-4.
Aligning AI’s Color Perception with Humans
Through their behavioral methods, the researchers discovered that adaptive techniques, particularly MCMC and Gibbs sampling, effectively mirror human-like color representations within GPT-4. This alignment between AI’s and humans’ conceptualizations of color highlights the potential of these methodologies to accurately probe and understand the internal representations of LLMs. It opens doors for further research into AI cognition and its parallels with human cognitive processes.
Implications Beyond Color Perception
While the study primarily focuses on color perception, its implications extend far beyond this specific realm. By employing cognitive psychology methods, researchers can gain insights into various aspects of AI cognition. Understanding how AI models perceive and process information has significant implications for natural language understanding, problem-solving, and reasoning tasks. By unraveling the black box of AI models, researchers can pave the way for advancements in the field and enhance the capabilities of these systems.
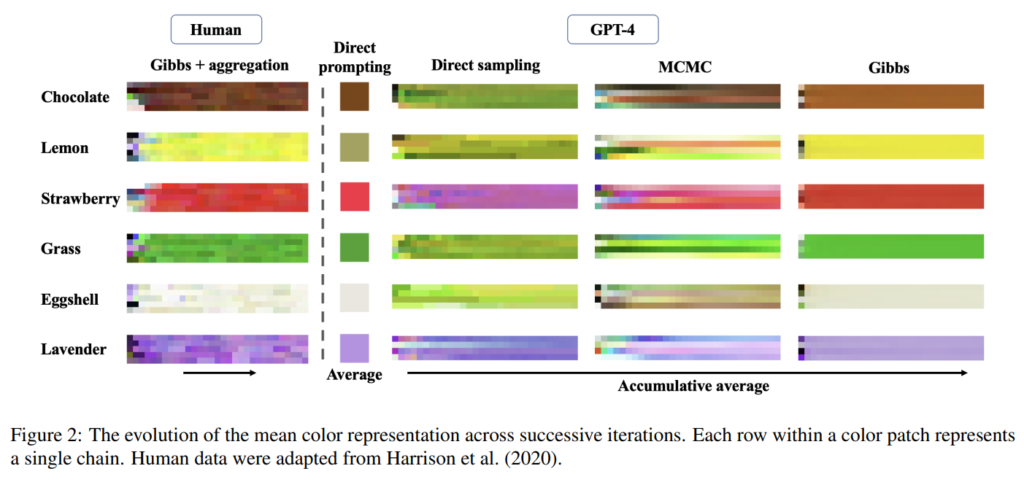
Conclusion
The study on decoding the color perception of Large Language Models (LLMs) through cognitive psychology methods represents a significant step toward unraveling the inner workings of AI systems. By employing direct sampling, MCMC, and other techniques, researchers have gained insights into GPT-4’s mental representations of color. This interdisciplinary approach not only sheds light on AI cognition but also offers potential avenues for further research and advancements in the field of artificial intelligence.
Understanding how AI models perceive and process information is crucial for enhancing their capabilities and developing more advanced systems. By bridging the gap between cognitive psychology and AI research, we can decipher the complexities of AI cognition and unlock new possibilities for future technologies.
Check out the Paper. All credit for this research goes to the researchers of this project. Also, don’t forget to follow us on LinkedIn. Do join our active AI community on Discord.
If you like our work, you will love our Newsletter 📰