Exploring the Frontier of AI Efficiency: Compressing Language Models Without Compromising Accuracy
Language models have revolutionized many applications by harnessing the power of human language. These models enable translation, content creation, and conversational AI breakthroughs. However, their enormous size presents challenges in terms of computational requirements and environmental impact. To address these issues, researchers from Seoul National University have conducted a survey exploring the frontier of AI efficiency by compressing language models without compromising accuracy.
The Delicate Balance: Model Size and Performance
Enhancing language model efficiency requires finding the delicate balance between model size and performance. Earlier models were engineering marvels capable of understanding and generating human-like text. However, their operational demands restricted their accessibility and raised concerns about their long-term viability and environmental impact. To address this conundrum, researchers have developed innovative techniques aimed at slimming down language models without diluting their capabilities.
Pruning and Quantization: Key Techniques for Efficiency
Two key techniques for compressing language models are pruning and quantization. Pruning involves identifying and removing parts of the model that contribute little to its performance. By reducing the model’s size and complexity, pruning leads to gains in efficiency. Quantization simplifies the model’s numerical precision, effectively compressing its size while maintaining its essential characteristics. These techniques represent a potent arsenal for creating more manageable and environmentally friendly language models.
Comprehensive Survey of Optimization Techniques
The survey conducted by researchers from Seoul National University delves into the depths of optimization techniques for language models. It presents a comprehensive survey that spans the gamut from high-cost, high-precision methods to innovative, low-cost compression algorithms. These low-cost compression algorithms are particularly noteworthy as they offer hope for making large language models more accessible.
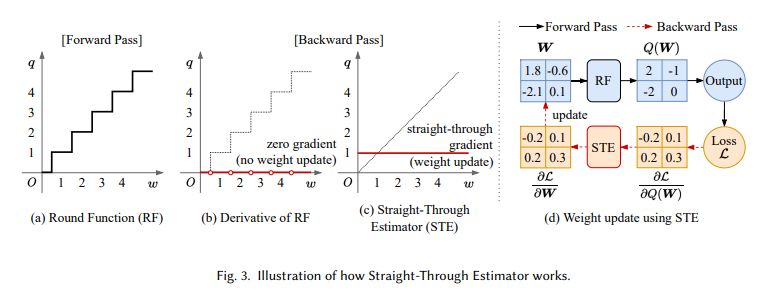
Democratizing Access to Advanced AI Capabilities
Low-cost compression algorithms significantly reduce the size and computational demands of large language models. This reduction in size and complexity promises to democratize access to advanced AI capabilities. By making language models more accessible, these compression techniques have the potential to drive progress and foster inclusivity across various applications.
Surprising Efficacy of Low-Cost Compression Algorithms
The survey reveals the surprising efficacy of low-cost compression algorithms in enhancing model efficiency. These previously underexplored methods have shown remarkable promise in reducing the footprint of large language models without compromising performance. The study’s in-depth analysis of these techniques highlights their unique contributions and underscores their potential as a focal point for future research.
Implications and Future Directions
The implications of this research extend far beyond the immediate benefits of reduced model size and improved efficiency. By paving the way for more accessible and sustainable language models, these optimization techniques have the potential to catalyze further innovations in AI. They promise a future where advanced language processing capabilities are within reach of a broader array of users, fostering inclusivity and driving progress across various applications.
Conclusion
The quest to optimize language models requires a relentless balance between size and performance, accessibility, and capability. The study conducted by researchers from Seoul National University sheds light on innovative compression techniques that unlock the full potential of language models. As we stand on the brink of this new frontier, the possibilities are as vast as the digital universe. The pursuit of more efficient, accessible, and sustainable language models is not only a technical challenge but also a gateway to a future where AI is interwoven into our daily lives, enhancing our capabilities, and enriching our understanding of the world.
Check out the Paper. All credit for this research goes to the researchers of this project. Also, don’t forget to follow us on LinkedIn. Do join our active AI community on Discord.
If you like our work, you will love our Newsletter 📰