Google DeepMind Researchers Propose a Novel AI Method Called Sparse Fine-grained Contrastive Alignment (SPARC) for Fine-Grained Vision-Language Pretraining
In recent years, there has been a growing interest in developing artificial intelligence (AI) models that can understand and generate human-like language. These models, known as vision-language models, have shown promising results in a wide range of tasks, including image classification, object detection, and image captioning. However, there is still room for improvement when it comes to fine-grained tasks such as localization and spatial relationships.
To address this challenge, researchers from Google DeepMind have proposed a novel AI method called Sparse Fine-grained Contrastive Alignment (SPARC) for fine-grained vision-language pretraining [1]. SPARC focuses on learning groups of image patches corresponding to individual words in captions, enabling detailed information capture in a computationally efficient manner.
The Need for Fine-grained Vision-Language Pretraining
Contrastive pre-training using large, noisy image-text datasets has become popular for building general vision representations. These models align global image and text features in a shared space through similar and dissimilar pairs, excelling in tasks like image classification and retrieval. However, they often struggle with fine-grained tasks that require a deeper understanding of the visual content.
🔥Explore 3500+ AI Tools and 2000+ GPTs at AI Toolhouse
While recent efforts have incorporated losses between image patches and text tokens to capture finer details, challenges such as computational expense and reliance on pre-trained models still persist. SPARC aims to address these issues and improve the performance of vision-language models in fine-grained tasks.
How SPARC Works
SPARC introduces a sparse similarity metric to compute language-grouped vision embeddings for each token in the caption. This approach allows for the capture of detailed information while remaining computationally efficient. The method combines fine-grained sequence-wise loss with a contrastive loss, enhancing performance in both coarse-grained tasks like classification and fine-grained tasks like retrieval, object detection, and segmentation.
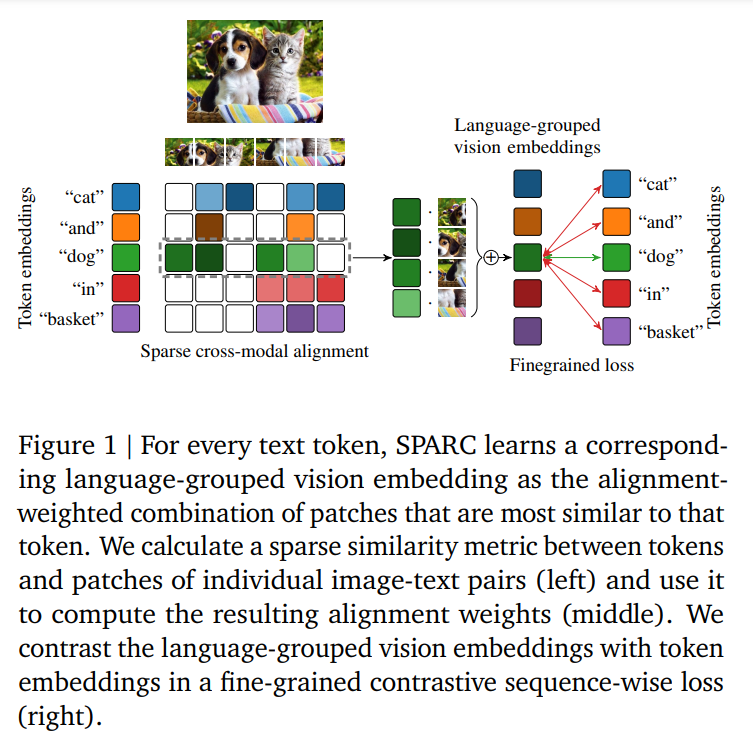
To achieve fine-grained vision-language pretraining, SPARC focuses on learning groups of image patches corresponding to individual words in captions. By aligning these patches with the language tokens, the model can capture detailed information about the visual content. The sparse similarity metric ensures that the computations are performed in a computationally efficient manner, making SPARC suitable for large-scale datasets.
The combination of fine-grained sequence-wise loss and contrastive loss allows SPARC to encode both global and local information simultaneously. This approach improves the overall performance of vision-language models, making them more capable of handling fine-grained tasks.
Advantages of SPARC
SPARC offers several advantages over existing methods for vision-language pretraining. Firstly, it improves the model’s ability to understand and generate fine-grained visual content. By explicitly modeling the relationship between image patches and language tokens, SPARC captures detailed information that is often overlooked by other approaches.
Secondly, SPARC enhances model faithfulness and captioning in foundational vision-language models. By incorporating fine-grained contrastive alignment, SPARC aligns the visual and textual components more effectively, resulting in more accurate and coherent captions.
Furthermore, SPARC achieves superior performance in both image-level tasks like classification and region-level tasks such as retrieval, object detection, and segmentation. The combination of fine-grained sequence-wise loss and contrastive loss enables the model to capture both coarse and fine-grained visual features, leading to improved results across a wide range of tasks.
Comparison with Existing Approaches
While SPARC is a novel method for fine-grained vision-language pretraining, it is important to compare it with existing approaches to understand its unique contributions.
CLIP and ALIGN are two popular contrastive image-text pre-training methods that leverage textual supervision from large-scale data scraped from the internet. These models excel in building general visual representations but often struggle with fine-grained understanding. SPARC addresses this limitation by explicitly modeling the relationship between image patches and language tokens, enabling it to capture fine-grained details more effectively.
FILIP, another approach, proposes a cross-modal late interaction mechanism to optimize the token-wise maximum similarity between image and text tokens. While this helps in addressing the problem of coarse visual representation in global matching, SPARC goes a step further by incorporating fine-grained sequence-wise loss and contrastive loss, leading to improved performance in fine-grained tasks.
PACL, on the other hand, starts from CLIP-pre trained vision and text encoders and trains an adapter through a contrastive objective to improve fine-grained understanding. While PACL focuses on fine-grained understanding, SPARC extends its capabilities by incorporating sparse similarity metrics and fine-grained sequence-wise loss. This combination allows SPARC to capture detailed information in a computationally efficient manner.
GLoRIA is another approach that builds localized visual representations by contrasting attention-weighted patch embeddings with text tokens. However, GLoRIA becomes computationally intensive for large batch sizes. In contrast, SPARC’s sparse similarity metric and fine-grained loss enable efficient training even on large-scale datasets.
Evaluation and Future Directions
The SPARC study evaluated its performance across various tasks, including image-level tasks like classification and region-level tasks such as retrieval, object detection, and segmentation. It outperformed existing methods in both task types, demonstrating its effectiveness in improving model performance.
Additionally, the study conducted zero-shot segmentation by comparing patch embeddings of an image with text embeddings of ground-truth classes. This evaluation method allowed for the assessment of SPARC’s ability to accurately segment objects in an image. The results showed promising improvements in accuracy compared to other approaches.
Looking ahead, SPARC opens the door for further advancements in fine-grained vision-language pretraining. By incorporating sparse similarity metrics and fine-grained loss, researchers can continue to refine the model’s performance and explore its applications in various domains.
Conclusion
Google DeepMind’s SPARC is a novel AI method that addresses the need for fine-grained vision-language pretraining. By leveraging sparse fine-grained contrastive alignment, SPARC enables detailed information capture in a computationally efficient manner. This method enhances the performance of vision-language models in both coarse-grained and fine-grained tasks, outperforming existing approaches.
With its unique contributions and advantages, SPARC has the potential to drive significant progress in the field of vision-language understanding. As researchers continue to explore its applications and refine its capabilities, we can expect further breakthroughs in AI models’ ability to understand and generate human-like language based on visual content.
Check out the Paper. All credit for this research goes to the researchers of this project. Also, don’t forget to follow us on LinkedIn. Do join our active AI community on Discord.
If you like our work, you will love our Newsletter 📰