Harmonizing Vision and Language: The Advent of Bi-Modal Behavioral Alignment (BBA) in Enhancing Multimodal Reasoning
Integrating vision and language has long been a challenge in the field of artificial intelligence. While significant progress has been made in individual domains, the true potential lies in the harmonious alignment of these two modalities. This is where the advent of Bi-Modal Behavioral Alignment (BBA) comes into play. BBA is a novel approach that enhances multimodal reasoning by bridging the gap between vision and language, unlocking a new realm of possibilities for complex reasoning tasks.
The Challenge of Multimodal Reasoning
Multimodal reasoning is the process of combining information from multiple modalities, such as visual and textual input, to arrive at a meaningful understanding or decision. It is a critical capability for AI models that aim to interact with the digital world in a more nuanced and human-like manner. However, achieving seamless integration between different modalities is not an easy task.
Traditional approaches to multimodal reasoning often face challenges when dealing with the complexities of professional and intricate domains. The inherent differences between visual and textual representations pose a significant hurdle, requiring innovative solutions to bridge the gap and leverage the strengths of both modalities.
The Advent of Bi-Modal Behavioral Alignment
Researchers from The University of Hong Kong and Tencent AI Lab have introduced the Bi-Modal Behavioral Alignment (BBA) method to address the challenges of multimodal reasoning. BBA is a prompting strategy meticulously designed to align the reasoning processes of large vision-language models (LVLMs) by harmonizing vision and language representations.
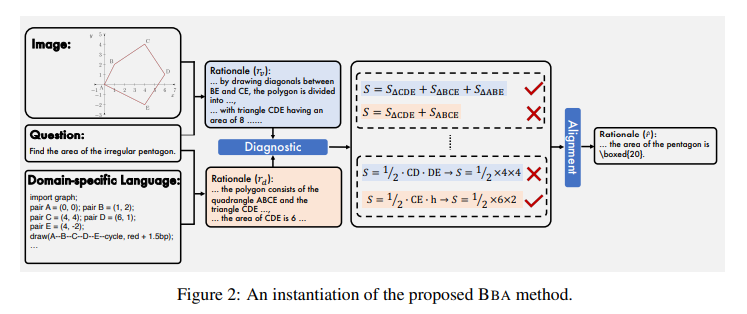
The BBA method starts by prompting LVLMs to generate separate reasoning chains for each modality, focusing on visual and domain-specific language (DSL) representations. This separation allows for a more fine-grained analysis of the reasoning steps and facilitates the identification of discrepancies between the two chains. The next step involves aligning these chains by reconciling the discrepancies, ensuring a cohesive integration of vision and language.
Leveraging the Strengths of Vision and Language
BBA utilizes a late fusion strategy to maintain the unique advantages of direct vision input and DSL representation. By treating inconsistencies across modalities as beneficial signals, BBA identifies and emphasizes critical steps within the reasoning process, enhancing the model’s ability to navigate complex reasoning tasks with unprecedented precision.
The integration of vision and language through BBA brings forth a synergistic relationship. Visual cues provide an intuitive grasp of the problem space, while DSL representations offer precision and context-specific knowledge. By combining these modalities, BBA enables LVLMs to leverage the best of both worlds, resulting in enhanced performance and accuracy across a variety of multimodal reasoning tasks.
Empirical Evidence and Future Exploration
BBA has been evaluated extensively across a range of multimodal reasoning tasks, including geometry problem solving, chess positional advantage prediction, and molecular property prediction. The results have been promising, showcasing significant performance improvements compared to traditional approaches.
In geometry problem solving, BBA has demonstrated a remarkable leap in performance, highlighting its versatility and adaptability across diverse domains. The empirical evidence, backed by rigorous comparative analysis, reaffirms the effectiveness of BBA in harnessing the synergies between vision and DSL representations.
The research on BBA not only addresses the fundamental challenges of integrating disparate reasoning mechanisms but also sets a new benchmark for accuracy and efficiency in complex reasoning tasks. It opens up avenues for further exploration and refinement in the field of artificial intelligence.
Conclusion
The advent of Bi-Modal Behavioral Alignment (BBA) represents a significant stride in enhancing multimodal reasoning capabilities. By harmonizing vision and language through a meticulous alignment process, BBA unlocks the true potential of large vision-language models (LVLMs) in tackling complex reasoning tasks.
The strategic fusion of vision and language in BBA leverages the unique strengths of both modalities, resulting in improved performance and accuracy. The empirical evidence from various multimodal reasoning tasks validates the effectiveness of BBA in enhancing LVLMs’ reasoning capabilities.
As we continue to unravel the intricate tapestry of human cognition through the lens of artificial intelligence, BBA stands as a milestone, paving the way for a future where AI’s potential knows no bounds. The integration of vision and language through BBA not only enriches our understanding of multimodal reasoning but also propels us toward new frontiers in AI research and development.
Check out the Paper. All credit for this research goes to the researchers of this project. Also, don’t forget to follow us on LinkedIn. Do join our active AI community on Discord.
If you like our work, you will love our Newsletter 📰