Researchers from UCLA and Snap Introduce Dual-Pivot Tuning: A Groundbreaking AI Approach for Personalized Facial Image Restoration
Image restoration is a challenging task in the field of artificial intelligence. The goal is to restore degraded or damaged images to their original quality, while also preserving the unique features of the subject. Researchers from the University of California, Los Angeles (UCLA), and Snap Inc. have recently introduced a groundbreaking AI approach called Dual-Pivot Tuning for personalized facial image restoration. This innovative technique aims to enhance the accuracy and fidelity of the restoration process by incorporating personalized prior information.
The Challenge of Personalized Facial Image Restoration
In traditional image restoration techniques, the focus has been on achieving general restoration results that apply to a wide range of images. However, when it comes to restoring facial images, a personalized approach is necessary to ensure that the output reflects the individual’s unique facial features. Traditional methods often struggle to preserve the identity of the person in the restored images, leading to a loss of fidelity and accuracy.
To address this challenge, the researchers from UCLA and Snap introduced Dual-Pivot Tuning, an AI-based approach that leverages personalized prior information for facial image restoration. The technique involves fine-tuning a text-to-image prior using a limited set of high-quality images of the individual, thereby enhancing the restoration process for their other degraded images.
The Dual-Pivot Tuning Approach
The Dual-Pivot Tuning technique comprises two main steps: text-based fine-tuning and model-centric pivoting. In the first step, a text-to-image diffusion model is fine-tuned using a limited set of high-quality images as a pivot. This fine-tuning process embeds identity-specific information within the diffusion priors, enabling the model to better capture the unique facial features of the individual.
The second step, model-centric pivoting, focuses on harmonizing the guiding image encoder with the personalized priors. This process ensures that the restoration process maintains a natural appearance while preserving the individual’s identity. By combining these two steps, the Dual-Pivot Tuning technique achieves high-fidelity restoration results that accurately reflect the person’s unique facial features.
Advantages and Significance of Dual-Pivot Tuning
The introduction of Dual-Pivot Tuning represents a significant breakthrough in the field of personalized facial image restoration. Unlike traditional diffusion-based blind restoration methods, Dual-Pivot Tuning can effectively preserve the individual’s identity in the restored images. By leveraging personalized priors, the technique achieves high identity fidelity without any perceivable loss in fidelity to the degraded input.
The researchers conducted qualitative and quantitative evaluations to assess the effectiveness of the Dual-Pivot Tuning technique. Qualitative comparisons demonstrated that diffusion-based blind restoration approaches often fail to retain the individual’s identity. In contrast, the proposed technique consistently maintains high identity fidelity while producing natural-looking restored images.
Quantitative evaluations using metrics such as Peak Signal-to-Noise Ratio (PSNR), Structural Similarity (SSIM), and ArcFace similarity further validated the superiority of the Dual-Pivot Tuning technique. The results showed that the proposed method outperforms various state-of-the-art alternatives for blind and few-shot personalized face image restoration. The customized model not only shows improved fidelity to the person’s identity but also ensures superior general image quality compared to generic priors.
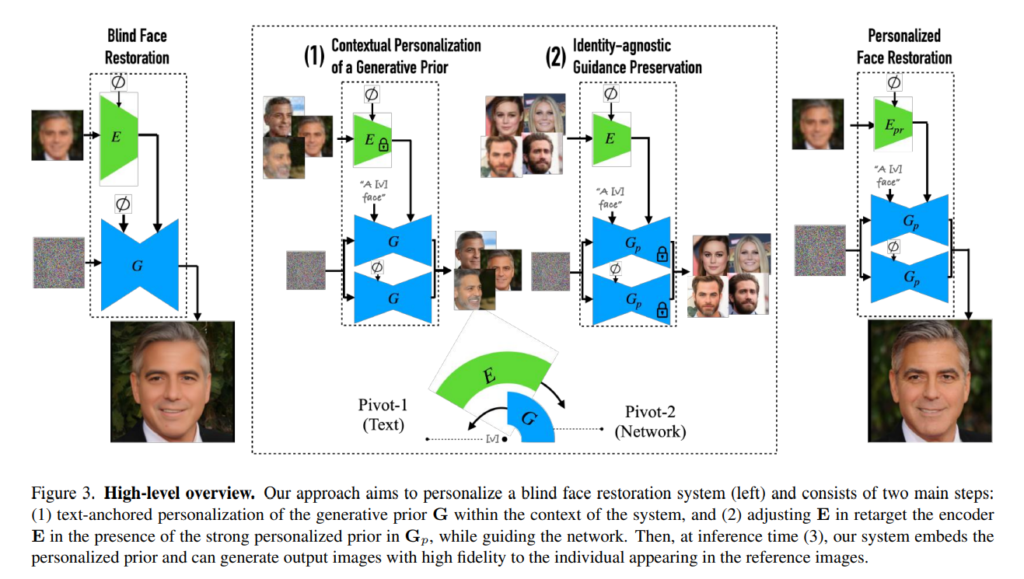
Applications and Future Implications
The Dual-Pivot Tuning technique opens up new possibilities in the field of personalized facial image restoration. With its ability to accurately restore the unique features of an individual’s face, this approach has potential applications in various domains like photography, forensics, and digital image processing.
In addition to its immediate practical implications, the introduction of Dual-Pivot Tuning also contributes to the advancement of AI research. By addressing the challenge of personalized restoration, this technique brings us closer to achieving AI systems that can truly understand and replicate the intricacies of human facial features.
The researchers from UCLA and Snap continue to refine and enhance the Dual-Pivot Tuning technique. Future developments may involve expanding the method to handle different types of image degradation and exploring its potential application in other image-related tasks beyond facial restoration.
Conclusion
The introduction of Dual-Pivot Tuning represents a groundbreaking AI approach for personalized facial image restoration. This technique addresses the challenge of preserving the individual’s identity while restoring degraded images. By leveraging personalized priors and incorporating fine-tuning and model-centric pivoting, Dual-Pivot Tuning achieves high identity fidelity and natural appearance in the restored images.
The significance of this technique lies not only in its practical applications but also in its contribution to the field of AI research. Dual-Pivot Tuning brings us one step closer to developing AI systems that can accurately restore and replicate human facial features. With further advancements and research, this approach has the potential to revolutionize the field of image restoration and pave the way for new possibilities in AI-driven image processing.