LLaVA-NeXT: Advancements in Multimodal Understanding and Video Comprehension
Multimodal understanding, the ability to comprehend and analyze information from different modalities such as text, images, and videos, is a crucial aspect of artificial intelligence. With the rapid growth of multimedia data on the internet, there is a pressing need for advanced models that can effectively process and understand these multimodal inputs. LLaVA-NeXT is a groundbreaking model that represents a significant advancement in the field of multimodal understanding and video comprehension. In this article, we will explore the key advancements and features of LLaVA-NeXT and delve into its impact on AI research and applications.
Understanding LLaVA-NeXT
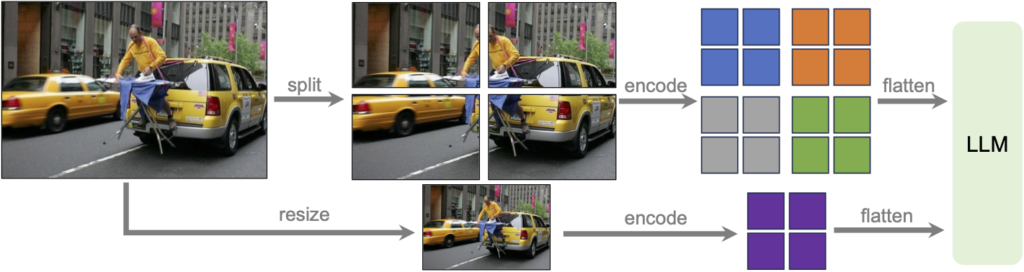
LLaVA-NeXT, developed by researchers from Nanyang Technological University, University of Wisconsin-Madison, and Bytedance, is an open-source multimodal model trained solely on text-image data. It builds upon the success of previous models like LLaVA (Large Language and Vision Assistant) and LLaVA-1.5, aiming to achieve a deeper understanding of multimodal data and enhance video comprehension capabilities.
Advancements in Multimodal Understanding
LLaVA-NeXT introduces several key advancements in multimodal understanding, leveraging the innovative AnyRes technique. AnyRes enhances reasoning, Optical Character Recognition (OCR), and world knowledge, resulting in exceptional performance across various image-based multimodal tasks. By surpassing benchmarks like MMMU and MathVista, LLaVA-NeXT demonstrates its effectiveness in multimodal understanding tasks.
One of the notable achievements of LLaVA-NeXT is its zero-shot video representation capability. It exhibits an unprecedented ability to transfer knowledge from text-image inputs to videos, enabling LLMs (Language and Vision Models) to comprehend and analyze video content. This advancement opens up new possibilities for video comprehension and analysis in AI applications.
Robust Video Comprehension
LLaVA-NeXT showcases robust performance in video comprehension through various key enhancements. Leveraging the AnyRes technique, it overcomes token length constraints and efficiently handles longer videos by employing linear scaling techniques. This allows the model to capture comprehensive video content and generalize its understanding across a wide range of video sequences.
Supervised fine-tuning (SFT) and direct preference optimization (DPO) further enhance LLaVA-NeXT’s video understanding prowess. SFT involves training the model with labeled video data, enabling it to learn and improve its comprehension abilities through supervised learning. DPO leverages the feedback generated by LLMs to optimize LLaVA-NeXT-Video, resulting in significant performance gains. These techniques contribute to LLaVA-NeXT’s state-of-the-art performance in video comprehension tasks.
Efficient Deployment and Scalability
In addition to its impressive performance, LLaVA-NeXT offers efficient deployment and scalability through the SGLang framework. SGLang enables 5x faster inference, making it suitable for applications that require high-speed processing of video data, such as million-level video re-captioning. This feature enhances the practicality and usability of LLaVA-NeXT in real-world scenarios.
Comparison with Other Models
LLaVA-NeXT’s advancements in multimodal understanding and video comprehension position it as a strong contender against other proprietary models like Gemini-Pro. It surpasses Gemini-Pro on benchmarks like MMMU and MathVista, highlighting its superior performance in multimodal tasks. The ability of LLaVA-NeXT to transfer knowledge from text-image inputs to videos also sets it apart from other models, as it enables zero-shot video representation and comprehension.
Future Prospects and Implications
The advancements in multimodal understanding and video comprehension brought by LLaVA-NeXT have significant implications for AI research and applications. By enabling AI models to effectively process and comprehend multimodal inputs, LLaVA-NeXT opens up new possibilities for applications in various domains, including image and video captioning, multimodal dialogue systems, and more.
The AnyRes algorithm used in LLaVA-NeXT provides a flexible framework for efficient processing of high-resolution images and videos. This algorithm, coupled with length generalization techniques and direct preference optimization, showcases promising prospects for refining training methodologies in multimodal contexts. These advancements may lead to further breakthroughs in multimodal understanding and enhance the performance and applicability of AI models in real-world scenarios.
Conclusion
LLaVA-NeXT represents a significant advancement in multimodal understanding and video comprehension. Its innovative AnyRes technique, coupled with advancements in length generalization, supervised fine-tuning, and direct preference optimization, enables LLaVA-NeXT to achieve exceptional performance in multimodal tasks. With its zero-shot video representation capability and efficient deployment through SGLang, LLaVA-NeXT showcases its versatility and scalability in handling multimodal data.
The advancements brought by LLaVA-NeXT have far-reaching implications for AI research and applications, and they open up new possibilities for analyzing and comprehending multimedia data. As the field of multimodal understanding continues to evolve, LLaVA-NeXT stands as a remarkable model that pushes the boundaries of AI capabilities and brings us closer to achieving Artificial General Intelligence.
Explore 3600+ latest AI tools at AI Toolhouse 🚀. Don’t forget to follow us on LinkedIn. Do join our active AI community on Discord.
Read our other blogs on AI Tools 😁
If you like our work, you will love our Newsletter 📰