Meet TinyLLaVA: The Game-Changer in Machine Learning with Smaller Multimodal Frameworks Outperforming Larger Models
Large multimodal models (LMMs) have been at the forefront of machine learning, revolutionizing how machines interpret and understand human languages and visual information. These models have the potential to offer more intuitive and natural ways for machines to interact with our world. However, one of the challenges in multimodal learning is accurately interpreting and synthesizing information from both textual and visual inputs. This process is complex due to the need to understand the distinct properties of each modality and effectively integrate these insights into a cohesive understanding.
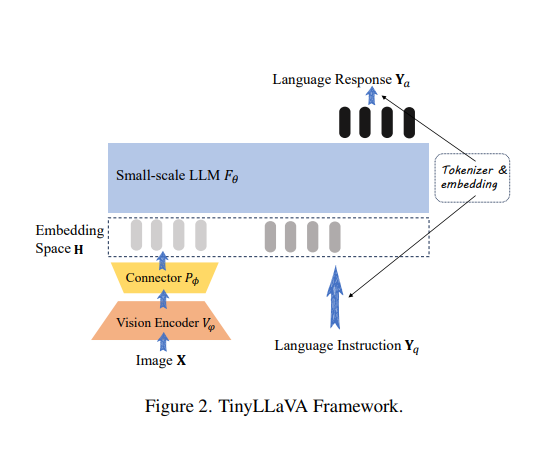
In recent years, extensive research has focused on autoregressive LLMs for vision-language learning. The aim is to effectively exploit LMMs by treating visual signals as conditional information. Researchers are also exploring methods to fine-tune LMMs with visual instruction tuning data to enhance their zero-shot capabilities. While large-scale LMMs have shown promising results, there is a growing interest in developing smaller multimodal frameworks that can achieve comparable performance while reducing computational overhead.
Introducing TinyLLaVA: A Framework for Small-scale Multimodal Models
To address the need for smaller multimodal frameworks, researchers from Beihang University and Tsinghua University in China have introduced TinyLLaVA . TinyLLaVA is a novel framework that utilizes small-scale LMMs for multimodal tasks. The framework comprises a vision encoder, a small-scale LLM decoder, an intermediate connector, and tailored training pipelines.
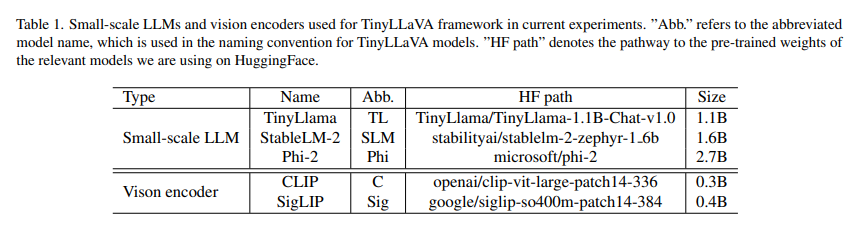
The primary goal of TinyLLaVA is to achieve high performance in multimodal learning while minimizing computational demands. It achieves this by training a family of small-scale LMMs and identifying the best-performing model. The research demonstrates that the top-performing model, TinyLLaVA-3.1B, outperforms existing 7B models such as LLaVA-1.5 and Qwen-VL. This remarkable outcome showcases the potential of smaller LMMs when optimized with suitable data and training methodologies.
Training and Performance of TinyLLaVA
The training process of TinyLLaVA involves combining vision encoders like CLIP-Large and SigLIP with small-scale LMMs to achieve better performance. The researchers utilized two different datasets, LLaVA-1.5 and ShareGPT4V, to study the impact of data quality on LMM performance. The framework allows the adjustment of partially learnable parameters of the LLM and vision encoder during the supervised fine-tuning stage.
Through a series of experiments, the researchers made significant findings. Model variants employing larger LMMs and the SigLIP vision encoder demonstrated superior performance. Additionally, the shared recipe, which includes vision encoder fine-tuning, enhanced the effectiveness of all model variants. Notably, the TinyLLaVA-share-Sig-Phi variant, with 3.1B parameters, outperformed the larger 7B parameter LLaVA-1.5 model in comprehensive benchmarks.
The Advantages of TinyLLaVA in Multimodal Learning
TinyLLaVA represents a significant step forward in multimodal learning. By leveraging small-scale LMMs, the framework offers a more accessible and efficient approach to integrating language and visual information. The development of TinyLLaVA enhances our understanding of multimodal systems and opens up new possibilities for their application in real-world scenarios.
One of the key advantages of TinyLLaVA is its ability to achieve impressive performance while maintaining reasonable computing budgets. This is particularly important in practical applications where computational resources may be limited. The smaller footprint of TinyLLaVA models allows for faster training times, lower memory requirements, and reduced energy consumption without compromising on performance.
Furthermore, the framework provides a unified analysis of model selections, training recipes, and data contributions to the performance of small-scale LMMs. This holistic approach allows researchers and practitioners to gain insights into the underlying factors that contribute to the success of multimodal learning. It also opens up avenues for further research and improvement in the field.
Conclusion
TinyLLaVA is a game-changer in machine learning, introducing smaller multimodal frameworks that outperform larger models while minimizing computational demands. By leveraging small-scale LMMs, the framework offers an accessible and efficient approach to integrating language and visual information. The success of TinyLLaVA underscores the importance of innovative solutions in advancing the capabilities of artificial intelligence. With further research and development, these smaller multimodal frameworks have the potential to revolutionize how machines interact with our world, offering more intuitive and natural ways for machines to understand and respond to human languages and visual information.
Check out the Paper. All credit for this research goes to the researchers of this project. Also, don’t forget to follow us on LinkedIn. Do join our active AI community on Discord.
If you like our work, you will love our Newsletter 📰